Data Collaboration Explained
There are multiple paths to privacy-first digital media targeting, and many depend on successful data collaboration. So what are the best ways for marketers to work with trusted data partners to pursue their targeted marketing objectives?
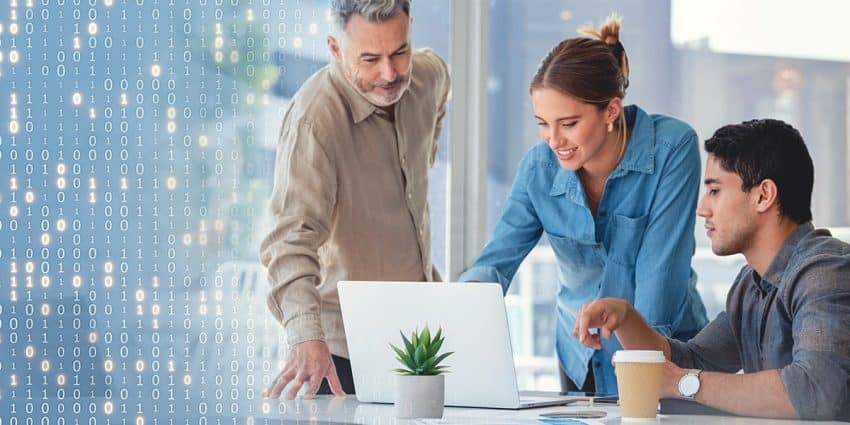
Data collaboration is not a new concept in marketing. Cooperative databases have been utilized by marketers to expand their acquisition capabilities for more than 30 years. It is a solution that has evolved to meet the needs of digital advertisers as well as direct mailers, and the use of AI-enabled response prediction technology has grown increasingly sophisticated and effective as well.
In today’s digital advertising environment of evolving data privacy regulations and the deprecation of third-party cookies and mobile advertising IDs, data collaboration takes on even more significance and importance. Collaborating with a trusted data partner is how digital marketers can find a path forward and leverage a larger scope of high-quality, permissioned data to fuel optimal marketing results. It should be noted here that data collaboration does not mean data sharing. In fact, data collaboration is founded on the non-movement of data. Instead, it is based on the privacy-compliant analysis of multiple data sets to obtain insights not available to just one source.
There are three types of data collaboration that are proving particularly successful with forward-thinking U.S. brands. These are data cooperatives, data marketplaces and exchanges, and technical data environments. Here is a quick summary of how each of these approaches work:
- Data Cooperatives
When brands and organizations participate in a data cooperative (also known as a cooperative database), they contribute their first-party data assets into a second-party data environment that is able to securely access all records as appropriate. This massive data is then analyzed by sophisticated predictive modeling systems to identify the high-value customers, donors, or subscribers who are most likely to be responsive to the marketing of the participants.A cooperative database operates on a “give to get” model that enables organizations to benefit from a comprehensive, cross-category pool of first-party data. The most effective approach to cooperative databases is the blind cooperative, in which contributing organizations do not have visibility into who the cooperative’s other contributors are nor the details of their data. A prime example of this is the Wiland Cooperative Database, which holds and analyzes the data of thousands of clients across categories covering $1 trillion+ in spending of over 250 million U.S. adult consumers, along with billions of other purchase intent and social media engagement signals.
Cooperative databases not only help organizations discover large universes of highly responsive prospects, but can also—when they have sufficiently advanced capabilities—offer valuable insights and optimize customer marketing campaigns. By maintaining strict permissions and privacy controls, a cooperative database enables participating organizations to future-proof their targeted digital advertising.
- Data Marketplaces and Exchanges
According to the Winterberry Group report Collaborative Data Solutions: Data and Identity in the Era of Permission, the rise of data marketplaces—which are also often referred to as data exchanges—can be directly attributed to the need for privacy-compliant digital media targeting. Within a data marketplace, data is securely activated by its owners directly into third-party targeting solutions or other analytics systems, all while protecting the privacy and integrity of the information. This means that marketers can use or license consumer data attributes, based on predetermined permissions, for the targeting and measurement of their advertising campaigns.The types of digital data attributes that populate data marketplaces have become more useful and reliable over the years. What were once directional online signals built around loose observations, such as page views and “likes”, are now highly predictive audiences that can be built using actual spending data, making them more reliably predictive of future spending behavior.
As we anticipate the final deprecation of the third-party cookies that have long powered addressable marketing (even as Google recently announced a further delay to this phase-out), powerful open-source identity solutions will continue to enable effective data marketplaces and exchanges. These systems provide secure, compliant identity solutions in an era of a fragmented and increasingly strict patchwork of federal and state privacy statutes.
- Technical Data Environments
Often referred to as “data clean rooms,” technical data environments provide extremely high levels of privacy and security in data partnerships. Evolving from the merge/purge systems that have securely supported direct mail marketers for decades, technical data environments take the time-tested concept of a neutral third-party data facilitator and automates it for users. In a technical data environment, both data provider and data recipient upload their consumer data in their own terms (with their own record ID values). The technical data environment is then able to securely match these two data “universes” on a diverse range of attributes. Records that can be matched are mapped, while non-matching records are discarded. This mapping powers two types of operations:- Aggregated Summary Data: The technical data environment uses the provider’s data to deliver reports and insights to the recipient without any consumer-level information. These are very powerful for formulating high-level strategy.
- Record-Level Provider Data: The technical data environment returns information to the recipient in their own terms (with their own record ID values—and only for records originally provided and therefore known by the provider) that allows understanding, selection, and exclusion of specific ad impression targeting. This is a powerful form of secure, anonymous addressable advertising.
This approach can be adapted to support different data collaboration models and can be deployed to provide numerous capabilities, including “data environment as a service.” Technical data environments fundamentally excel at enabling the sharing of permissioned data between partners in a secure, privacy-centric way.
Doing this correctly requires substantial technical expertise and infrastructure. We expect to see further definition, refinement, and innovation in what has become a vital part of modern marketing stacks.
Amidst the challenges of today’s digital marketing ecosystem, data collaboration in all of its secure and appropriate forms is a proven yet ever-evolving methodology. It helps marketers engage individual consumers more effectively and accurately within proper privacy constraints. Brands and organizations need to be proactive about forming the necessary data partnerships that will drive their long-term success and even future-proof—as much as possible—their digital media targeting.
Tags: data collaboration data privacy digital advertising digital audiences first-party data second-party data third-party-data